Short-term forecasting of greenhouse tomato price before supply to the market: Isfahan-Iran
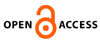
Department of Agricultural Extension and Education, College of Agriculture, Razi University, Kermanshah, Iran.
DOI: https://doi.org/10.37855/jah.2021.v23i01.16
Key words: Tomato price, price forecasting; artificial neural networks, support vector machines; efficiency comparison
Abstract: A reliable forecast of the prices of agricultural commodities can help to allocate resources optimally, enhance efficiency and farmer income, and alleviate fluctuations. Given the importance of accurate forecasts, the present paper investigates how one can forecast greenhouse tomato prices one, two, three, and six-month horizons using different support vector machines and artificial neural network algorithms. The data on variables affecting the price of greenhouse tomatoes were collected through a field study for a short-term period from November 2014 to January 2017. The wholesale price of the crop was drawn from a market study for this period. The results show that the trend forecasted through General Regression Neural Network along with activating function of reciprocal is more efficient to estimate the training data. The Epsilon-SVR SVM acquisition pattern alongside the linear activating function was taken into consideration to estimate the testing data in an efficient way for two-month, three-month or biannual periods.