Evaluating pre-trained CNNs for distinguishing fresh vs rotten fruits and vegetables
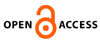
1Ludwig Maximilian University of Munich (LMU), Germany. 2Technical University of Munich (TUM), Germany. 3Aydin Adnan Menderes University (ADU), Turkey. Corresponding e-mail: serra.aksoy@campus.lmu.de
DOI: https://doi.org/10.37855/jah.2024.v26i03.68
Key words: MobileNetV3 Small, EfficientNetV2 Small, DenseNet121, ShuffleNetV2_x1_5, image classification, fruit and vegetable diseases
Abstract: Accurately distinguishing between fresh and rotten fruits and vegetables is essential for reducing waste, ensuring food safety, and maintaining quality standards in agriculture and supply chain management. This research utilized the fruit and vegetable diseases dataset from Kaggle, which included images of 14 types of produce in both healthy and rotten states. In this study, the performance of four pre-trained convolutional neural network models was evaluated: MobileNetV3 Small, EfficientNetV2 Small, DenseNet121, and ShuffleNetV2_x1_5. Among these, ShuffleNetV2_x1_5 demonstrated the highest performance, achieving an overall accuracy of 94.61% and a cross-validation mean accuracy of 96.43% with minimal standard deviation. The model’s efficiency and robust feature extraction capabilities make it highly suitable for real-time applications in agricultural monitoring and robotics, offering a significant advancement in the automation of produce quality assessment.